33.3. NP-Completeness¶
33.3.1. Hard Problems¶
There are several ways that a problem could be considered hard. For example, we might have trouble understanding the definition of the problem itself. At the beginning of a large data collection and analysis project, developers and their clients might have only a hazy notion of what their goals actually are, and need to work that out over time. For other types of problems, we might have trouble finding or understanding an algorithm to solve the problem. Understanding spoken English and translating it to written text is an example of a problem whose goals are easy to define, but whose solution is not easy to discover. But even though a natural language processing algorithm might be difficult to write, the program's running time might be fairly fast. There are many practical systems today that solve aspects of this problem in reasonable time.
None of these is what is commonly meant when a computer theoretician uses the word "hard". Throughout this section, "hard" means that the best-known algorithm for the problem is expensive in its running time. One example of a hard problem is Towers of Hanoi. It is easy to understand this problem and its solution. It is also easy to write a program to solve this problem. But, it takes an extremely long time to run for any "reasonably" large value of n. Try running a program to solve Towers of Hanoi for only 30 disks!
The Towers of Hanoi problem takes exponential time, that is, its running time is Θ(2n). This is radically different from an algorithm that takes Θ(nlogn) time or Θ(n2) time. It is even radically different from a problem that takes Θ(n4) time. These are all examples of polynomial running time, because the exponents for all terms of these equations are constants. If we buy a new computer that runs twice as fast, the size of problem with complexity Θ(n4) that we can solve in a certain amount of time is increased by the fourth root of two. In other words, there is a multiplicative factor increase, even if it is a rather small one. This is true for any algorithm whose running time can be represented by a polynomial.
Consider what happens if you buy a computer that is twice as fast and try to solve a bigger Towers of Hanoi problem in a given amount of time. Because its complexity is Θ(2n), we can solve a problem only one disk bigger! There is no multiplicative factor, and this is true for any exponential algorithm: A constant factor increase in processing power results in only a fixed addition in problem-solving power.
There are a number of other fundamental differences between polynomial running times and exponential running times that argues for treating them as qualitatively different. Polynomials are closed under composition and addition. Thus, running polynomial-time programs in sequence, or having one program with polynomial running time call another a polynomial number of times yields polynomial time. Also, all computers known are polynomially related. That is, any program that runs in polynomial time on any computer today, when transferred to any other computer, will still run in polynomial time.
There is a practical reason for recognizing a distinction. In practice, most polynomial time algorithms are "feasible" in that they can run reasonably large inputs in reasonable time. In contrast, most algorithms requiring exponential time are not practical to run even for fairly modest sizes of input. One could argue that a program with high polynomial degree (such as n100) is not practical, while an exponential-time program with cost 1.001n is practical. But the reality is that we know of almost no problems where the best polynomial-time algorithm has high degree (they nearly all have degree four or less), while almost no exponential-time algorithms (whose cost is (O(cn)) have their constant c close to one. So there is not much gray area between polynomial and exponential time algorithms in practice.
For the purposes of this Module, we define a hard algorithm to be one that runs in exponential time, that is, in Ω(cn) for some constant c>1. A definition for a hard problem will be presented soon.
33.3.2. The Theory of NP-Completeness¶
Imagine a magical computer that works by guessing the correct solution from among all of the possible solutions to a problem. Another way to look at this is to imagine a super parallel computer that could test all possible solutions simultaneously. Certainly this magical (or highly parallel) computer can do anything a normal computer can do. It might also solve some problems more quickly than a normal computer can. Consider some problem where, given a guess for a solution, checking the solution to see if it is correct can be done in polynomial time. Even if the number of possible solutions is exponential, any given guess can be checked in polynomial time (equivalently, all possible solutions are checked simultaneously in polynomial time), and thus the problem can be solved in polynomial time by our hypothetical magical computer. Another view of this concept is this: If you cannot get the answer to a problem in polynomial time by guessing the right answer and then checking it, then you cannot do it in polynomial time in any other way.
The idea of "guessing" the right answer to a problem—or checking all possible solutions in parallel to determine which is correct—is a called a non-deterministic choice. An algorithm that works in this manner is called a non-deterministic algorithm, and any problem with an algorithm that runs on a non-deterministic machine in polynomial time is given a special name: It is said to be a problem in NP. Thus, problems in NP are those problems that can be solved in polynomial time on a non-deterministic machine.
Not all problems requiring exponential time on a regular computer are in NP. For example, Towers of Hanoi is not in NP, because it must print out O(2n) moves for n disks. A non-deterministic machine cannot "guess" and print the correct answer in less time.
It turns out that there is a large collection of problems with this property: We know efficient non-deterministic algorithms, but we do not know if there are efficient deterministic algorithms. At the same time, we have not been able to prove that any of these problems do not have efficient deterministic algorithms. This class of problems is called NP-complete. What is truly strange and fascinating about NP-complete problems is that if anybody ever finds the solution to any one of them that runs in polynomial time on a regular computer, then by a series of reductions, every other problem that is in NP can also be solved in polynomial time on a regular computer!
Define a problem to be NP-hard if any problem in NP can be reduced to X in polynomial time. Thus, X is as hard as any problem in NP. A problem X is defined to be NP-complete if
- X is in NP, and
- X is NP-hard.
terms of Figure 33.3.1.
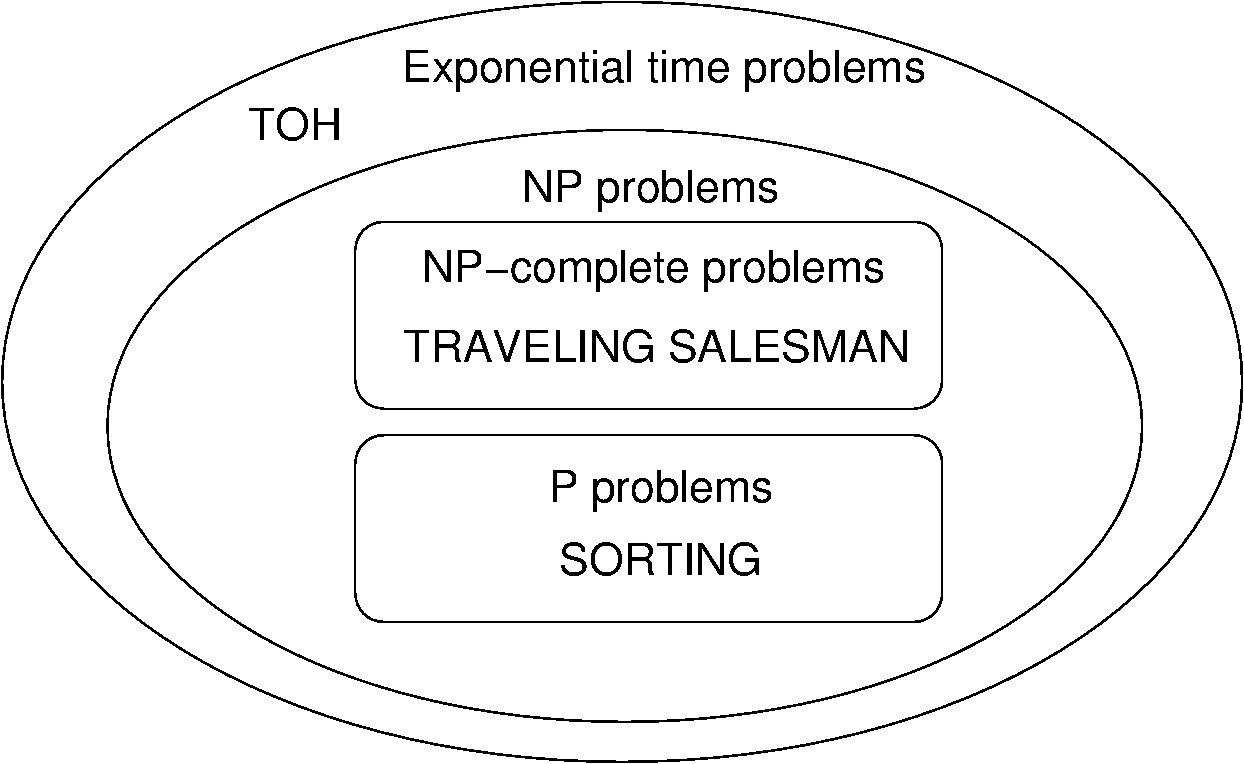
Figure 33.3.1: Our knowledge regarding the world of problems requiring exponential time or less. Some of these problems are solvable in polynomial time by a non-deterministic computer. Of these, some are known to be NP-complete, and some are known to be solvable in polynomial time on a regular computer.
The most important unanswered question in theoretical computer science is whether P=NP.
The next few modules show examples of NP Complete Problems.